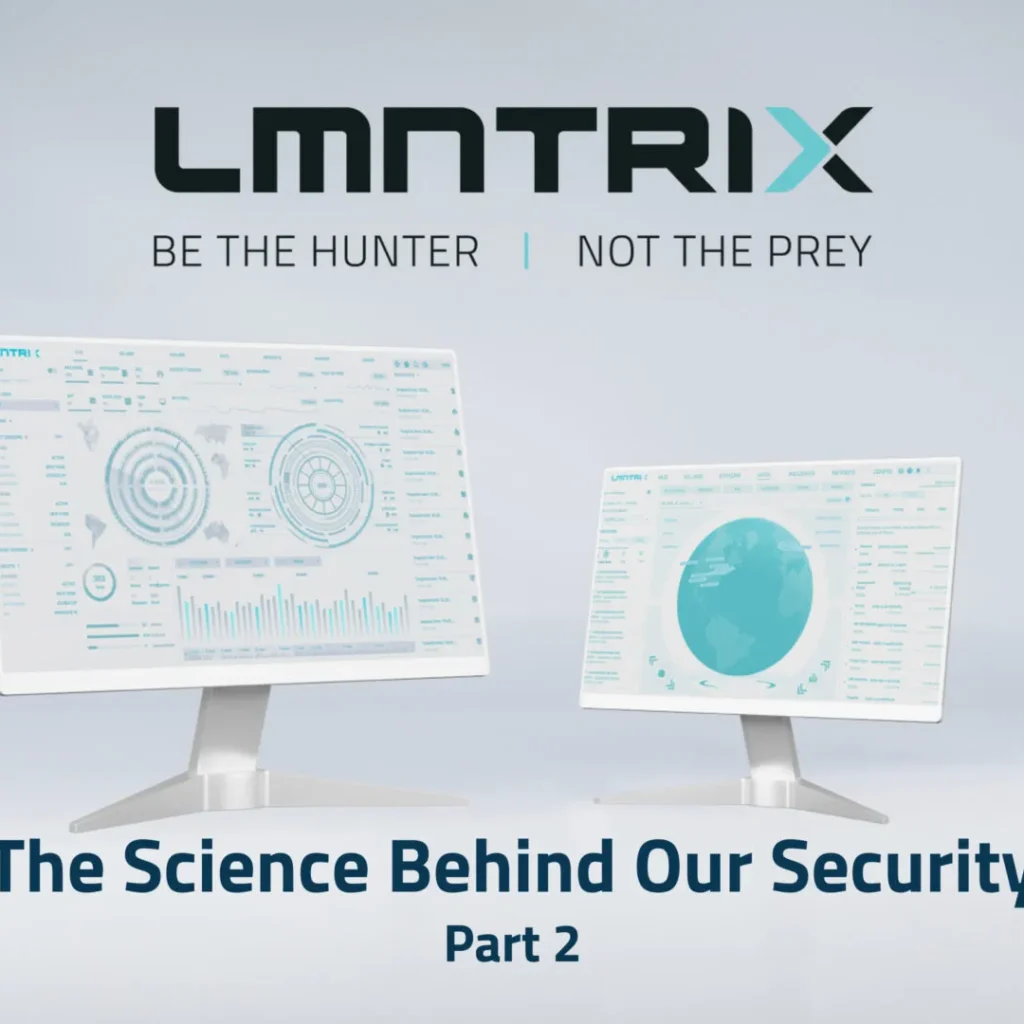
In our first blog on the “Science Behind our Security”, we talked about the three pillars: Models, MBIs, and the Attack Sequence. In this blog, we will focus on the machine learning models. These models are a true differentiator for LMNTRIX. I know, everyone says this, but in this blog, we will back it up.
LMNTRIX has an amazing data science team – if you have watched our webinars or videos, you know I have raved about them. This team has taken machine learning models to the next level. Our solution has three levels of machine learning models to contextualize activities, events, and behaviors so that when your security team is alerted – you know it is an alert worth your while. This extensive analysis also means that you will not be overwhelmed with false positives and researching “alerts” that are a complete waste of the security team’s time.
Machine Learning Models Across the Cloud, for the Cloud, and in the Cloud
It is difficult for security teams to determine if a behavior, activity, or event is malicious or a threat, or just a one-off behavior. The data science team builds machine learning models at several layers within the environment, so you are only responding to actual threats:
LMNTRIX XDR Platform: This is an aggregate view of risk across all our customers’ clouds, for roles and assets within the cloud. The models at this level provide a very wide view of context and help assess the overall risk of the attack sequence. To learn more about our attack sequence, check out our previous blog on The Science Behind our Security.
The Customer Cloud: Several models are created to detect threatening behaviors or events within each customer cloud. For example, models that are built to detect suspicious or malicious behaviors in the network.
Users and Cloud Assets. Finally, the Data Science team creates models for users, roles, assets, and functions to look for suspicious network traffic or API usage.
The output from these models is correlated and contextualized. Many events are mapped into single malicious behavior indicators, which are then correlated into an attack sequence (learn more here). The models at the LMNTRIX XDR platform helps assess the level of risk, sequence and once a threshold has reached, they are raised to an alert. This is an unprecedented level of context that gives security teams the utmost confidence that the alerts they are responding to are real alerts and require attention while eliminating false positives and preserving the productivity of the team.
The data science team reviews and updates these models on a daily basis. This is extremely powerful for two important reasons:
The daily updates eliminate drift ensuring the models are accurate. The models are always effective and up to date on the latest regular behaviors in the environment ensuring that the alerts that are raised in the environment are real.
Daily updates mean threat actors cannot go around, outrun, or avoid LMNTRIX models. Threat actors cannot anticipate or reverse-engineer our models because they change every day.
Summary
LMNTRIX machine learning models are different because they are created for so many levels in the environment, to model so many different behaviors. These models are updated on a daily basis so threat actors cannot reverse-engineer these models and avoid them. The daily updates ensure threat actors cannot outrun LMNTRIX XDR.